10. Cloud and Edge Architectures
Chapter Objectives
- 10-1 Differentiate between centralized, decentralized, and distributed networks.
- 10-2 Explain the role of edge computing and endpoint devices in effective data transmission.
- 10-3 Describe cloud and edge networking and their major use cases.
- 10-4 Identify the major challenges in cloud and edge networking.
- 10-5 Explain the role of fog computing in a cloud and edge infrastructures.
Centralized, Decentralized, and Distributed Networks
In our introduction to network models, we described two models of networking models: the client-server and peer-to-peer models. These networks describe how nodes communicate with each other. Centralized, decentralized, and distributed network models describe how data and control are distributed among the nodes. We describe these three models below.
A centralized network model is one where data and control are concentrated in one node or a group of nodes that have the authority to make decisions for the whole network. A centralized network model is simple, consistent, and reliable, but it has low fault-tolerance, scalability, and autonomy. Centralized models provide organizations with greater control over their activities, faster decision-making, greater consistency, improved efficiencies, and accountability. However, they also have disadvantages, such as less flexibility, less innovation, and security risks.
A decentralized network model is one where data and control are distributed among multiple nodes or groups of nodes. Each node or group of nodes has some authority to make decisions for itself or its group. A decentralized network model is resilient, scalable, and autonomous, but it has higher complexity, inconsistency, and coordination costs. A decentralized network typically operates on a peer-to-peer architecture, with each node having equal decision-making power and no central supremacy. The absence of a global clock allows nodes to operate independently, while multiple central units enable the distribution of tasks and workloads. Decentralized networks provide characteristics such as load balancing among nodes, high availability, and enhanced autonomy over sources and information. The fault tolerance of decentralized systems enables continuous functioning even if nodes fail, while the transparent and open structure promotes accountability and trust. Enhanced security is achieved through the absence of a single point of failure and the distribution of data across multiple nodes. Scalability is improved by the ability to distribute the workload and accommodate high traffic volumes. However, decentralized systems face difficulties in identifying failed or responding nodes, regulatory oversight, resource utilization inefficiencies, lack of standardization, and slower transaction processing compared to their centralized counterparts.
A distributed network model is one where data and control are dispersed among many independent nodes. A distributed system represents a network configuration that comprises multiple independently run networks, fostering collaboration between nodes for data sharing and achieving shared objectives. Combining elements of client/server and peer-to-peer architecture, distributed systems facilitate the seamless communication and synchronization of data while mitigating bottlenecks and single points of failure. Each node has equal authority to make decisions for itself and cooperate with other nodes. A distributed network model is fault-tolerant, efficient, and flexible, but it may have challenges in coordination, consistency, and security.
Notable examples of distributed networks include applications in electronic banking, healthcare, internet giants like Google, multiplayer games, and cellular networks. These systems emphasize the exchange of data, applications, and computing tasks across interconnected nodes to achieve a collective goal. Key characteristics of distributed systems include resource sharing, simultaneous processing capabilities, scalability, error detection mechanisms, and node transparency, ensuring efficient and reliable network operation.
Distributed systems offer several advantages, including reduced latency due to their geographically dispersed nature, seamless scalability through the addition of new nodes, and robust fault tolerance allowing for continuous functionality even in the event of node failures. Enhanced reliability, cost-effectiveness through the utilization of off-the-shelf components, and improved performance via parallel processing techniques further contribute to their appeal. Despite these benefits, challenges exist within distributed systems, such as the difficulty in achieving consensus among nodes, complex network infrastructure requirements leading to potential network outages and data loss, security vulnerabilities, synchronization issues causing data conflicts, high development and maintenance costs, and limitations in scalability due to technical constraints and synchronization challenges. These complexities highlight the intricate nature of distributed systems and the need for careful management and implementation to ensure their effectiveness and stability.
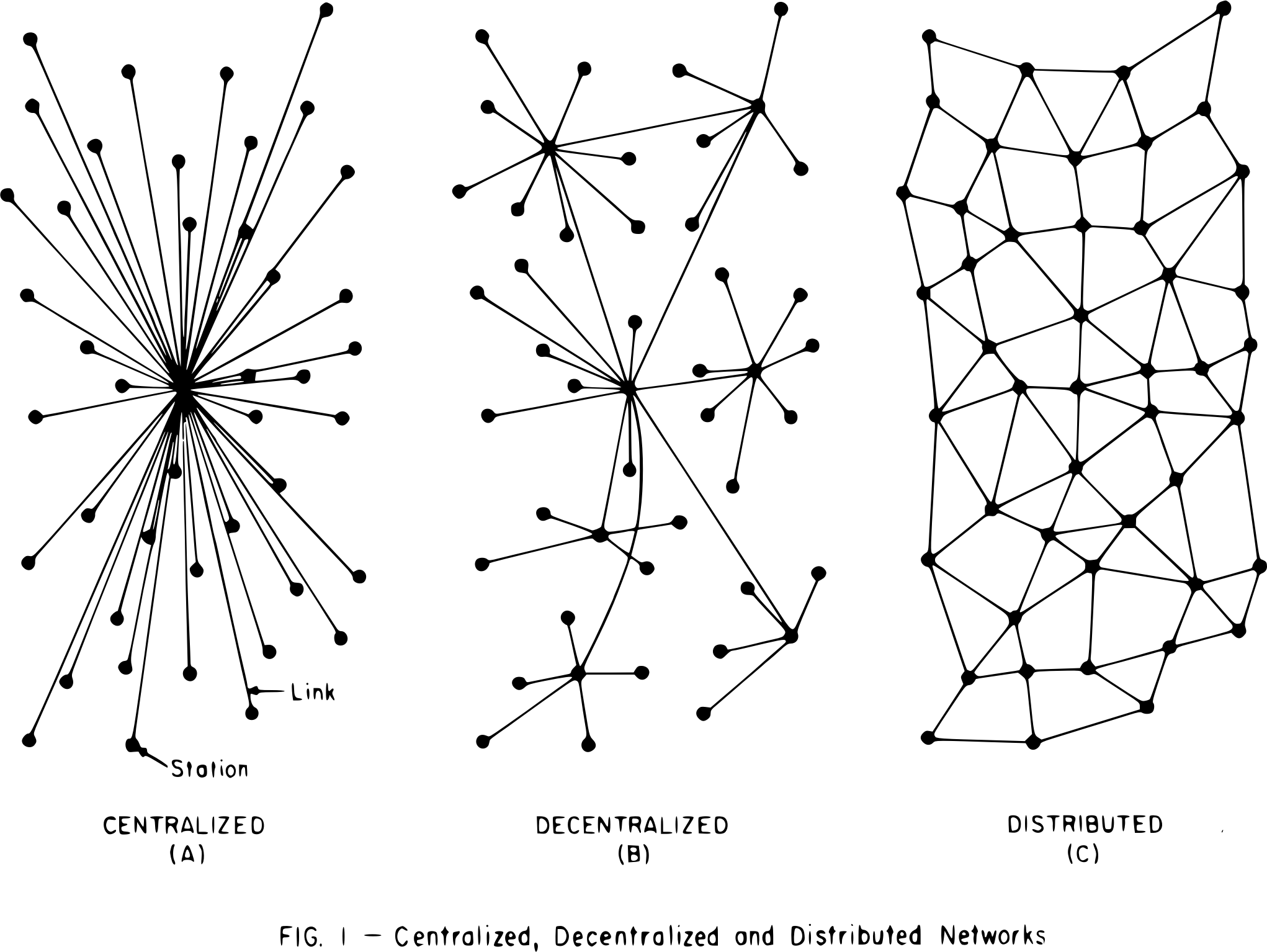
Cloud and Edge Networks
A cloud edge network is a type of distributed system. Cloud computing involves the delivery of computing services—including servers, storage, databases, networking, software, analytics, and intelligence—over the Internet (“the cloud”) to offer faster innovation, flexible resources, and economies of scale. It typically relies on a distributed network of servers located in data centers around the world. The key characteristic of cloud computing is the ability to provide on-demand resources and services over the internet with the help of a network of remote servers.
Edge computing is a distributed computing paradigm that brings computation and data storage closer to the location where it is needed, improving response times and saving bandwidth. Edge computing aims to process data on the edge of the network, near the source of the data. This can reduce latency and improve the overall performance of the system.
Both cloud and edge computing involve the distribution of computing resources and services across a network of devices and servers. This distributed nature allows for improved performance, scalability, and fault tolerance, similar to other types of distributed systems. While they have specific characteristics and functionalities that differentiate them from traditional distributed systems, they share the fundamental concept of leveraging a network of interconnected devices and servers to deliver computing resources and services to end-users.
For many years, centralized cloud computing has been considered a standard IT delivery platform. Though cloud computing is ubiquitous, emerging requirements and workloads are beginning to expose its limitations. With its strong data center centric view, where compute and storage resources are relatively plentiful and centralized, little or no thought was ever given to the optimization of the supporting hypervisor and management platform footprint. Few cloud developers seriously considered the requirements needed to support resource-constrained nodes reachable only over unreliable or bandwidth-limited network connections, or thought about the needs of applications that demand very high bandwidth, low latency, or widespread compute capacity across many sites.
New applications, services, and workloads increasingly demand a different kind of architecture, one that’s built to directly support a distributed infrastructure. New requirements for availability and cloud capability at remote sites are needed to support both today’s requirements (retail data analytics, network services) and tomorrow’s innovations (smart cities, AR/VR). The maturity, robustness, flexibility, and simplicity of cloud now needs to be extended across multiple sites and networks in order to cope with evolving demands.
Recently companies have begun to apply the simplified administration and flexibility of cloud computing architectures to distributed infrastructures that span across multiple sites and networks. Organizations have an emerging need to take cloud capabilities across WAN networks and into increasingly smaller deployments out at the network edge. Though this approach is in its early days, it is becoming clear that many emerging use cases and scenarios would benefit from distributed architectures.
The paper referenced in this chapter by the OSF Edge Computing Group explores this emerging need. This type of computing has been called many names: distributed cloud, fog computing, fourth generation data centers, but for the purposes of this document, we will stick with a common, easily understood term—cloud edge computing.
The OSF Edge Computing Group has taken up the challenge to describe fundamental requirements of a fully functional edge computing cloud infrastructure. In this chapter, we discuss their aims, which aim to accomplish several important tasks:
- Cultivate a conversation around cloud edge computing, including some basic definitions, stimulating interest and engagement from the open source community.
- Guide both the broader open source and OpenStack communities in developing tools and standards needed for broad adoption.
- Explore how current tools, standards and architectures may need to change to accommodate this distributed cloud model.
Concepts Corner
What is the difference between cloud computing and network computing? Is the Cloud a WAN?
It is important to understand the differences and similarities between networking and cloud computing. Networking refers to the connection or interaction of devices for communication. Cloud computing refers to the delivery of computing services over the Internet. As cloud computing services increase, the lines between networking and cloud computing blur due to the similarities as well as the services Cloud Service Providers (CSPs) may offer, both networking and cloud computing services.
Any time you have computers sharing resources, file sharing, application sharing, or printer sharing, you have a LAN (Local Area Network). A LAN can be one of many types of networks, such as a peer-to-peer network, and may be connected via wired (with cable or cabling) or wireless (via a router, no cables) networking. Definitions of WAN (Wide Area Network) may start with the number of computers. Some definitions use distance as a factor; however, neither the number of computers nor the distance between them is a factor. A WAN is more than one LAN connected together using a public or private connection. A WAN is considered a distributed private telecommunications network that interconnects multiple local area networks. A bank is an example of a WAN with offices, branches, and ATMs across a wide area, city, country, or continent. The Internet itself may be considered the largest public Wide Area Network as it is global covering the Earth and available on every continent inhabited.
A Metropolitan Area Network (MAN), may connect LANs within the city, or a smaller geographical area. A university is an example of a MAN connecting offices, buildings, and departments across an area larger than a LAN and smaller than a WAN.
According to Amazon Web Services (AWS), a wide area network is “the technology that connects your offices, data centers, cloud applications, and cloud storage together”. This may be seen as a new definition of WAN with the Cloud Services adding to the definition of WAN (data centers, cloud apps, and cloud storage). Cloud Services go beyond file sharing, printing, or applications. The Cloud Services now range from SAAS (software as a service), and IAAS (infrastructure as a service), to PAAS (platform as a service) and DAAS (databases as a service). All Cloud Services are available through the Cloud and an Internet connection.
Historically the features of a WAN included installation costs, maintenance or recurring costs to maintain, reliability, speed, as well as WAN design. These traditional WAN costs may now be assumed by Cloud Service Providers reducing or eliminating capital expenses to business owners. The bottom line, companies/businesses, do not need to spend the overhead to connect LANs, regionally, nationally, or internationally, they may move to the Cloud to reduce costs and benefit from Cloud reliability, scalability, as well as redundancy.
Wireless Fidelity Wi-Fi (IEEE 802.11x) is the wireless standard used in building wireless LANs while WiMax (Wireless Inter-operability for Microwave Access/(IEEE 802.16y) is primarily used by carriers in building wireless MANs. Wi-Fi is short-range (100 meters) while WiMax is used to establish connections at greater ranges (50-90 km.). WiMax is a bit antiquated as technologies have replaced most WiMax with or by LTE (long-term evolution) antennae or services.
A Cloud Service Provider (CSP) comparison begins with how AWS, Microsoft Azure, and Google Cloud are considered as Wide Area Networks (WANs). Cloud Service Providers (CSPs) include Amazon Web Services (AWS), Microsoft Azure, Google Cloud, IBM Cloud, and Alibaba (the list is not all-inclusive). These CSPs have worldwide data centers and distribution regions across the globe. These cloud service facilities in effect are a Wide Area Network offering voice, data, and image, transfers across and between countries to any user with a subscription to their product or site. A user, business, or organization is no longer required to build their own Wide Area Network, these entities may pay for the physically provided service. Two examples of Wide Area Network services are Azure Virtual WAN and AWS Cloud WAN. Both offer the ability to create private or public virtualized networks for an organization’s Cloud deployment. The associated capital expense costs of Wide Area Networks compared to Local Area Networks and Metropolitan Area Networks have been reduced or eliminated by the CSP services.
What is Edge Computing?
It is worth highlighting that many overlapping and sometimes conflicting definitions of edge computing exist—edge computing means many things to many people. But for our purposes, the most mature view of edge computing is that it is offering application developers and service providers cloud computing capabilities, as well as an IT service environment at the edge of a network.
The aim is to deliver compute, storage, and bandwidth much closer to data inputs and/or end users. An edge computing environment is characterized by potentially high latency among all the sites and low and unreliable bandwidth—alongside distinctive service delivery and application functionality possibilities that cannot be met with a pool of centralized cloud resources in distant data centers. By moving some or all of the processing functions closer to the end user or data collection point, cloud edge computing can mitigate the effects of widely distributed sites by minimizing the effect of latency on the applications.
Edge computing first emerged by virtualizing network services over WAN networks, taking a step away from the data center. The initial use cases were driven by a desire to leverage a platform that delivered the flexibility and simple tools that cloud computing users have become accustomed to.
As new edge computing capabilities emerge, we see a changing paradigm for computing—one that is no longer necessarily bound by the need to build centralized data centers. Instead, for certain applications, cloud edge computing is taking the lessons of virtualization and cloud computing and creating the capability to have potentially thousands of massively distributed nodes that can be applied to diverse use cases, such as industrial IoT or even far-flung monitoring networks for tracking real time water resource usage over thousands, or millions, of locations.
Many proprietary and open source edge computing capabilities already exist without relying on distributed cloud—some vendors refer to this as “device edge.” Components of this approach include elements such as IoT gateways or NFV appliances. But increasingly, applications need the versatility of cloud at the edge, although the tools and architectures needed to build distributed edge infrastructures are still in their infancy. Our view is that the market will continue to demand better capabilities for cloud edge computing.
Edge computing capabilities include, but are not limited to:
- A consistent operating paradigm across diverse infrastructures.
- The ability to perform in a massively distributed (think thousands of global locations) environment.
- The need to deliver network services to customers located at globally distributed remote locations.
- Application integration, orchestration and service delivery requirements.
- Hardware management and scope.
- Limited or intermittent network connections.
- Methods to address applications with strict low latency requirements (AR/VR, voice, and so forth).
- Geofencing and requirements for keeping sensitive private data local.
A Deeper Exploration of Edge Computing Considerations
The “edge” in edge computing refers to the outskirts of an administrative domain, as close as possible to discrete data sources or end users. This concept applies to telecom networks, to large enterprises with distributed points of presence such as retail, or to other applications, in particular in the context of IoT.
One of the characteristics of edge computing is that the application is strongly associated with the edge location. For telecoms, “the edge” would refer to a point close to the end user but controlled by the provider, potentially having some elements of workloads running on end user devices. For large enterprises, “the edge” is the point where the application, service or workload is used (e.g. a retail store or a factory). For the purposes of this definition, the edge is not an end device with extremely limited capacity for supporting even a minimal cloud architecture, such as an IoT or sensor device. This is an important consideration, because many discussions of edge computing do not make that distinction.
Edge computing is similar to data center computing in that:
- It includes compute, storage and networking resources.
- Its resources may be shared by many users and many applications.
- It benefits from virtualization and abstraction of the resource pool.
- It benefits from the ability to leverage commodity hardware.
- It uses APIs to support interoperability.
Edge computing differs from computing in large data centers in that:
- Edge sites are as close as possible to end users to improve the experience over high latency and unreliable connections.
- Specialized hardware may be required, such as GPU/FPGA platforms for AR/VR functionality.
- Edge can scale to large numbers of sites, distributed in distinct locations.
- An edge site’s location and the identity of the access links it terminates are significant. An application that needs to run close to its users, needs to be in the right part of the edge. It is common for the application location to matter in edge computing.
- The entire pool of sites can be considered to be dynamic. Because of their physical separation, edge sites will, in some cases, be connected to each other and the core with WAN connections. Edge sites will join and leave the pool of infrastructure over time.
- Edge sites are remote and potentially unmanned, and therefore must be administered remotely. Tools need to support intermittent network access to the site.
- Edge supports large differences in site size and scale, from data center scale down to a single device.
- Edge sites may be resource constrained; adding capacity to an existing site is restricted due to space or power requirements.
- Multi-tenancy on a massive scale is required for some of the use cases.
- Isolation of edge computing from data center clouds may be required to ensure that compromises in the “external cloud” domain cannot impact services.
The concept of edge computing must cover both the edge site (e.g. the compute, network and storage infrastructure), but also the applications (workloads) that run on it. It is worth noting that any applications in an edge computing environment could potentially leverage any or all of the capabilities provided by a cloud—compute, block storage, object storage, virtual networking, bare metal, or containers.
The essential features that define and separate edge computing from cloud computing are as follows:
- The ability to support a dynamic pool of multiple potentially widely distributed sites,
- Potentially unreliable network connections, and
- the likelihood of difficult-to-resolve resource constraints at sites across the network.
Exploring Characteristics of Edge Computing
So what do we know so far about edge computing characteristics, use cases, and scenarios?
The defining need that drives cloud edge computing is the need for service delivery to be closer to users or end-point data sources. Edge computing environments will work in conjunction with core capacity, but aim to deliver an improved end user experience without putting unreasonable demands on connectivity to the core. Improvements result from:
- Reducing latency: The latency to the end user could be lower than it would be if the compute was farther away—making, for instance, responsive remote desktops possible, or successful AR, or better gaming.
- Mitigating bandwidth limits: The ability to move workloads closer to the end users or data collection points reduces the effect of limited bandwidth at a site. This is especially useful if the service on the edge node reduces the need to transmit large amounts of data to the core for processing, as is often the case with IoT and NFV workloads. Data reduction and local processing can be translated into both more responsive applications and reduces the cost of transporting terabytes of data over long distances.
But there are trade-offs. To deliver edge computing, it is necessary to vastly increase the number of deployments. This institutes a significant challenge to widespread edge deployments. If managing a single cloud takes a team of ten, how can an organization cope with hundreds or even thousands of small clouds? Some requirements include:
- Standardization and infrastructure consistency are needed. Each location has to be similar; a known quantity.
- Manageability needs to be automated; deployment, replacement and any recoverable failures should be simple and straightforward.
- Simple, cost-effective plans need to be laid for when hardware fails.
- Locally fault-tolerant designs might be important, particularly in environments that are remote or unreachable—zero touch infrastructure is desirable. This is a question that balances the cost of buying and running redundant hardware against the cost of outages and emergency repairs. Considerations include:
- Do these locations need to be self-sufficient?
- If a location has a failure, no one is going to be on-site to fix it, and local spares are unlikely.
- Does it need to tolerate failures? And if it does, how long is it going to be before someone will be available to repair it—two hours, a week, a month?
- Maintainability needs to be straightforward—untrained technicians perform manual repairs and replacements, while a skilled remote administrator re-installs or maintains software.
- Physical designs may need a complete rethink. Most edge computing environments won’t be ideal—limited power, dirt, humidity and vibration have to be considered.
Use Cases of Cloud and Edge Computing
There are probably dozens of ways to characterize use cases and this chapter is too short to provide an exhaustive list. But here are some examples to help clarify thinking and highlight opportunities for collaboration.
Four major categories of workload requirements that benefit from a distributed architecture are analytics, compliance, security, and NFV.
DATA COLLECTION AND ANALYTICS
IoT, where data is often collected from a large network of microsites, is an example of an application that benefits from the edge computing model. Sending masses of data over often limited network connections to an analytics engine located in a centralized data center is counterproductive; it may not be responsive enough, could contribute to excessive latency, and wastes precious bandwidth. Since edge devices can also produce terabytes of data, taking the analytics closer to the source of the data on the edge can be more cost-effective by analyzing data near the source and only sending small batches of condensed information back to the centralized systems. There is a tradeoff here—balancing the cost of transporting data to the core against losing some information.
SECURITY
Unfortunately, as edge devices proliferate––including mobile handsets and IoT sensors––new attack vectors are emerging that take advantage of the proliferation of endpoints. Edge computing offers the ability to move security elements closer to the originating source of attack, enables higher performance security applications, and increases the number of layers that help defend the core against breaches and risk.
COMPLIANCE REQUIREMENTS
Compliance covers a broad range of requirements, ranging from geofencing, data sovereignty, and copyright enforcement. Restricting access to data based on geography and political boundaries, limiting data streams depending on copyright limitations, and storing data in places with specific regulations are all achievable and enforceable with edge computing infrastructure.
NETWORK FUNCTION VIRTUALIZATION (NFV)
Network Function Virtualization (NFV) is at its heart the quintessential edge computing application because it provides infrastructure functionality. Telecom operators are looking to transform their service delivery models by running virtual network functions as part of, or layered on top of, an edge computing infrastructure. To maximize efficiency and minimize cost/complexity, running NFV on edge computing infrastructure makes sense.
REAL-TIME
Real-time applications, such as AR/VR, connected cars, telemedicine, tactile internet Industry 4.0 and smart cities, are unable to tolerate more than a few milliseconds of latency and can be extremely sensitive to jitter, or latency variation. As an example, connected cars will require low latency and high bandwidth, and depend on computation and content caching near the user, making edge capacity a necessity. In many scenarios, particularly where closed-loop automation is used to maintain high availability, response times in tens of milliseconds are needed, and cannot be met without edge computing infrastructure.
IMMERSIVE
Edge computing expands bandwidth capabilities, unlocking the potential of new immersive applications. Some of these include AR/VR, 4K video, and 360° imaging for verticals like healthcare. Caching and optimizing content at the edge is already becoming a necessity since protocols like TCP don’t respond well to sudden changes in radio network traffic. Edge computing infrastructure, tied into real-time access to radio/network information can reduce stalls and delays in video by up to 20% during peak viewing hours, and can also vary the video feed bitrate based on radio conditions.
NETWORK EFFICIENCY
Many applications are not sensitive to latency and do not require large amounts of nearby compute or storage capacity, so they could theoretically run in a centralized cloud, but the bandwidth requirements and/or compute requirements may still make edge computing a more efficient approach. Some of these workloads are common today, including video surveillance and IoT gateways, while others, including facial recognition and vehicle number plate recognition, are emerging capabilities. With many of these, the edge computing infrastructure not only reduces bandwidth requirements, but can also provide a platform for functions that enable the value of the application—for example, video surveillance motion detection and threat recognition. In many of these applications, 90% of the data is routine and irrelevant, so sending it to a centralized cloud is prohibitively expensive and wasteful of often scarce network bandwidth. It makes more sense to sort the data at the edge for anomalies and changes, and only report on the actionable data.
SELF-CONTAINED AND AUTONOMOUS SITE OPERATIONS
Many environments, even today, have limited, unreliable or unpredictable connectivity. These could include transportation (planes, buses, ships), mining operations (oil rigs, pipelines, mines), power infrastructure (wind farms, solar power plants), and even environments that should typically have good connectivity, like stores. Edge computing neatly supports such environments by allowing sites to remain semi-autonomous and functional when needed or when the network connectivity is not available. The best example of this approach is the need for retail locations to maintain their point of sales (POS) systems, even when there is temporarily no network connectivity.
PRIVACY
Enterprises may have needs for edge computing capacity depending on workloads, connectivity limits and privacy. For example, medical applications that need to anonymize personal health information (PHI) before sending it to the cloud could do this utilizing edge computing infrastructure.
Another way to look at requirements that would benefit from cloud edge computing is by the type of company that would deploy them. Operator applications are workloads put on edge computing infrastructure that is built and managed by operators—telecommunications companies, for example. Third-party applications are built by organizations to run on existing edge infrastructure, in order to leverage others’ edge computing infrastructure. It is worth noting that any applications could leverage any or all of the capabilities provided by a cloud—compute, block storage, object storage, virtual networking, bare metal, or containers.
SCENARIOS
The basic characteristic of the edge computing paradigm is that the infrastructure is located closer to the end user, that the scale of site distribution is high and that the edge nodes are connected by WAN network connections. Examining a few scenarios in additional depth helps us evaluate current capabilities that map to the use case, as well as highlighting weaknesses and opportunities for improvement.
1. Retail/finance/remote location “cloud in a box”: Edge computing infrastructure that supports a suite of applications customized to the specific company or industry vertical. Often used by the enterprise, edge computing infrastructure, ultimately coupled together into distributed infrastructure, to reduce the hardware footprint, standardize deployments at many sites, deliver greater flexibility to replace applications located at the edge (and to have the same application running uniformly in all nodes irrespective of HW), boost resiliency, and address concerns about intermittent WAN connections. Caching content or providing compute, storage, and networking for self-contained applications are obvious uses for edge computing in settings with limited connectivity.
2. Mobile connectivity: Mobile/wireless networks are likely to be a common environmental element for cloud edge computing, as mobile networks will remain characterized by limited and unpredictable bandwidth, at least until 5G becomes widely available. Applications such as augmented reality for remote repair and telemedicine, IoT devices for capturing utility (water, gas, electric, facilities management) data, inventory, supply chain and transportation solutions, smart cities, smart roads and remote security applications will all rely on the mobile network to greater or lesser degrees. They will all benefit from edge computing’s ability to move workloads closer to the end user.
3. Network-as-a-Service (NaaS): Coming from the need to deliver an identical network service application experience in radically different environments, the NaaS use case requires both a small footprint of its distributed platform at the edges, and strong centralized management tools that cross over unreliable or limited WAN network connections in support of the services out on the edge. The main characteristics of this scenario are: small hardware footprint, moving (changing network connections) and constantly changing workloads, hybrid locations of data and applications. This is one of the cases that needs infrastructure to support micro nodes—small doses of compute in non-traditional packages (not all 19 in rack in a cooled data center). NaaS will require support for thousands or tens of thousands of nodes at the edge and must support mesh and/or hierarchical architectures as well as on demand sites that might spin up as they are needed and shutdown when they are done. APIs and GUIs will have to change to reflect that large numbers of compute nodes will have different locations instead of being present in the same data center.
4. Universal Customer Premises Equipment (uCPE): This scenario, already being deployed today, demands support for appliance-sized hardware footprints and is characterized by limited network connections with generally stable workloads requiring high availability. It also requires a method of supporting hybrid locations of data and applications across hundreds or thousands of nodes and scaling existing uCPE deployments will be an emerging requirement.
This is particularly applicable to NFV applications where different sites might need a different set of service chained applications, or sites with a different set of required applications that still need to work in concert. Mesh or hierarchical architectures would need to be supported with localized capacity and the need to store and forward data processing due to intermittent network connections. Self-healing and self-administration combined with the ability to remotely administer the node are musts.
5. Satellite enabled communication (SATCOM): This scenario is characterized by numerous capable terminal devices, often distributed to the most remote and harsh conditions. At the same time, it makes sense to utilize these distributed platforms for hosting services, especially considering the extremely high latency, limited bandwidth and the cost of over-the-satellite communications. Specific examples of such use cases might include vessels (from fishing boats to tanker ships), aircrafts, oil rigs, mining operations or military grade infrastructure.
Challenges in Edge Computing
Though there are plenty of examples of edge deployments already in progress around the world, widespread adoption will require new ways of thinking to solve emerging and already existing challenges and limitations.
We have established that the edge computing platform has to be, by design, much more fault tolerant and robust than a traditional data center centric cloud, both in terms of the hardware as well as the platform services that support the application lifecycle. We cannot assume that such edge use cases will have the maintenance and support facilities that standard data center infrastructure does. Zero touch provisioning, automation, and autonomous orchestration in all infrastructure and platform stacks are crucial requirements in these scenarios.
But there are other challenges that need to be taken under consideration.
For one, edge resource management systems should deliver a set of high-level mechanisms whose assembly results in a system capable of operating and using a geo-distributed IaaS infrastructure relying on WAN interconnects. In other words, the challenge is to revise (and extend when needed) IaaS core services in order to deal with aforementioned edge specifics—network disconnections/bandwidth, limited capacities in terms of compute and storage, unmanned deployments, and so forth.
Some foreseeable needs include:
- A virtual-machine/container/bare-metal manager in charge of managing machine/container lifecycle (configuration, scheduling, deployment, suspend/resume, and shutdown).
- An image manager in charge of template files (a.k.a. virtual-machine/container images).
- A network manager in charge of providing connectivity to the infrastructure: virtual networks and external access for users.
- A storage manager, providing storage services to edge applications.
- Administrative tools, providing user interfaces to operate and use the dispersed infrastructure.
These needs are relatively obvious and could likely be met by leveraging and adapting existing projects. But other needs for edge computing are more challenging. These include, but are not limited to:
- Addressing storage latency over WAN connections.
- Reinforced security at the edge—monitoring the physical and application integrity of each site, with the ability to autonomously enable corrective actions when necessary.
- Monitoring resource utilization across all nodes simultaneously.
- Orchestration tools that manage and coordinate many edge sites and workloads, potentially leading toward a peering control plane or “self-organizing edge.”
- Orchestration of a federation of edge platforms (or cloud-of-clouds) has to be explored and introduced to the IaaS core services.
- Automated edge commission/decommission operations, including initial software deployment and upgrades of the resource management system’s components.
- Automated data and workload relocations—load balancing across geographically distributed hardware.
- Some form of synchronization of abstract state propagation should be needed at the “core” of the infrastructure to cope with discontinuous network links.
- New ways to deal with network partitioning issues due to limited connectivity—coping with short disconnections and long disconnections alike.
- Tools to manage edge application life cycles, including:
- The definition of advanced placement constraints in order to cope with latency requirements of application components.
- The provisioning/scheduling of applications in order to satisfy placement requirements (initial placement).
- Data and workload relocations according to internal/external events (mobility use-cases, failures, performance considerations, and so forth).
- Integration location awareness: Not all edge deployments will require the same application at the same moment. Location and demand awareness are a likely need.
- Discrete hardware with limited resources and limited ability to expand at the remote site needs to be taken into consideration when designing both the overall architecture at the macro level and the administrative tools. The concept of being able to grab remote resources on demand from other sites, either neighbors over a mesh network or from core elements in a hierarchical network, means that fluctuations in local demand can be met without inefficiency in hardware deployments.
Contributors
Discussion Topics
What are some of the security considerations in working with edge and fog networks?
- For Edge Computing
- Data Privacy and Security: Edge devices often handle sensitive data locally, making them vulnerable to unauthorized access and data breaches.
- Implement strong encryption protocols for data at rest and in transit, and use secure boot mechanisms and hardware-based security features to protect data integrity.
- Device Management and Authentication: Managing and authenticating a large number of edge devices can be challenging, leading to potential security gaps.
- Utilize robust identity and access management (IAM) systems, and implement multi-factor authentication (MFA) and regularly update device firmware to patch vulnerabilities.
- Distributed Denial of Service (DDoS) Attacks: Edge devices can be targeted by DDoS attacks, disrupting services and causing significant downtime.
- Use anomaly detection systems to identify and respond to unusual traffic patterns.
Fog Computing
- Data Integrity and Confidentiality: Fog nodes process and store data locally, which can be susceptible to tampering and unauthorized access.
- Implement end-to-end encryption and secure data storage solutions, with the potential use of blockchain technology to ensure data integrity and traceability.
- Resource Management and Isolation: Fog computing environments often share resources among multiple tenants, leading to potential security risks.
- Use virtualization and containerization technologies to isolate resources, and implement strict access controls and monitoring to prevent unauthorized resource usage.
- Physical Security of Fog Nodes: Fog nodes are often deployed in remote or less secure locations, making them vulnerable to physical tampering.
- Employ tamper-evident and tamper-resistant hardware, and use secure enclosures and physical access controls to protect fog nodes.
- Data Privacy and Security: Edge devices often handle sensitive data locally, making them vulnerable to unauthorized access and data breaches.